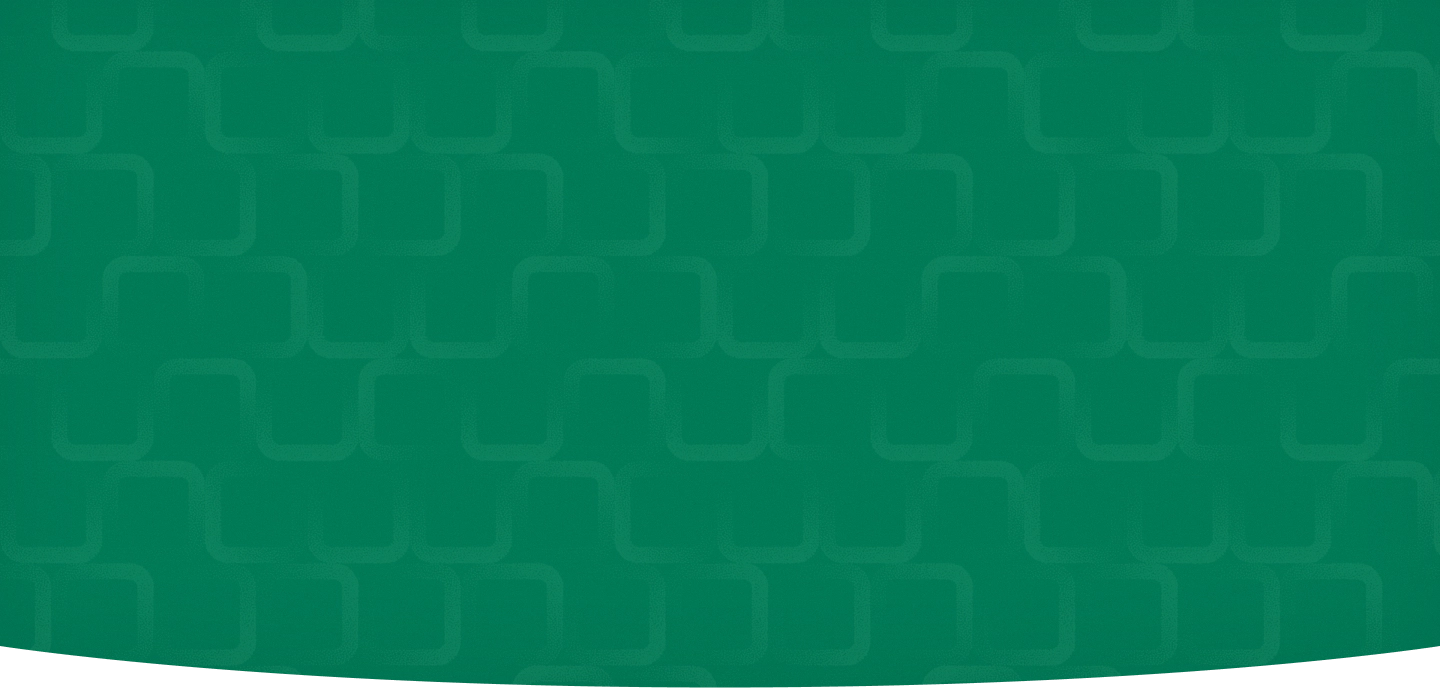
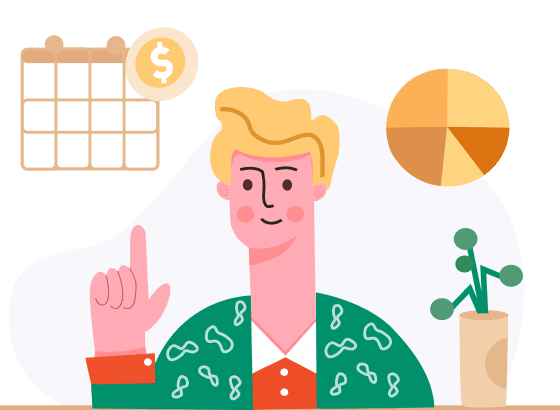
Sales forecasting shapes smarter decisions, turning data into a roadmap for success. With the right model, businesses can fine-tune inventory, staffing, and budgets, adapting swiftly to market shifts.
In 2025, the options are abundant, with different types of sales forecasting methods ranging from qualitative insights to time-based patterns and cause-driven analysis.
Let's explore eight sales forecasting methods that can help you stay ahead.
Three Categories of Sales Forecasting Models
Not all businesses forecast the same way. With different methods of sales forecasting, you can find what works best for you. To understand the “why” behind sales shifts, let's break down the three main methods of forecasting sales:
Qualitative Forecasting Methods
When raw data falls short, qualitative forecasting methods take over. They tap into human knowledge—expert insights, customer perspectives, and market research—to predict outcomes. Numbers alone can’t always capture the nuances of a new market, especially when launching something entirely new or when historical data is scarce.
While qualitative models can be powerful, they often come with a degree of subjectivity. What experts or customers predict can differ, and biases may color their forecasts. Nevertheless, they’re an essential tool when quantitative data simply isn’t enough. Think of qualitative forecasting as a way to guide your sales strategy when data alone doesn't paint the full picture.
Time Series Analysis
Time series forecasting draws its strength from historical data, looking at past trends to anticipate what’s ahead. It works on the simple idea that what happened before is likely to happen again. When your business moves to a familiar beat — whether it’s seasonal peaks or consistent sales spikes — this method can give you a reliable blueprint for what’s next.
Dive into your data, and you’ll see patterns that have a habit of showing up again and again. These insights let you anticipate what’s coming, so you can plan rather than react. It’s a way to use the past to help you stay two steps ahead of future surprises.
The challenge with time series forecasting is that it works best only when patterns remain consistent over time. If external conditions or market dynamics shift suddenly, the model’s predictions may not hold true. As such, it’s most effective for businesses in stable markets with predictable sales patterns.
Causal Forecasting Models
Causal forecasting goes beyond predicting future sales based on past patterns. This approach seeks to understand the causes or drivers of sales fluctuations. Instead of simply looking at data and predicting when sales will spike or dip, causal models investigate factors like marketing efforts, economic changes, or seasonal events that influence those trends.
Causal forecasting is like zooming in with a magnifying glass on what’s driving your sales numbers. Armed with a mix of internal insights (think marketing wins or product launches) and external factors like economic shifts, you’re able to make precise forecasts and adjust your game plan to dodge potential setbacks.
By understanding the ‘why’ behind the trends, causal models give you the power to plan with confidence
8 Sales Forecasting Models for Driving Business Growth
With so many methods of sales forecasting out there, it’s essential to pick the one that aligns with your business type and goals.
Here are eight popular sales forecasting techniques, both established and emerging, that can help take your sales forecasting to the next level.
1. Time Series Models
For businesses with predictable sales rhythms, time series forecasting makes planning straightforward. By drawing from past trends, this method helps you anticipate familiar spikes, like holiday shopping or end-of-quarter boosts, so you’re not caught off guard by cyclical demand.
The importance of sales forecasting becomes especially clear here, as understanding seasonal patterns and adjusting inventory ahead of time prevents both overstocking and stockouts.
How Time Series Works: This model relies on historical data—whether daily, weekly, monthly, or yearly—analyzing it to identify recurring patterns, such as seasonality, trends, or random fluctuations.
When to Use It: Time series forecasting excels when there are consistent, repeating sales patterns over time. For example, a clothing retailer could use time series models to anticipate increased demand during back-to-school or holiday periods, ensuring they stock the right amount of inventory ahead of these spikes.
2. Regression Analysis
Regression analysis helps identify relationships between sales and other variables like marketing spend, pricing, or consumer behavior. It highlights correlations that show how changes in one factor affect sales.
How Regression Works: It’s like putting together a puzzle, where you analyze both the things you control (like marketing spend) and what’s being affected (sales). The beauty of regression is that it gives you the “cause and effect” behind your sales numbers.
When to Use It: For those situations when several variables are affecting your sales – be it promotions or price, regression lets you realize which of them is significant. For a software company, it could reveal which marketing campaigns are generating the most subscriptions. Now you know where you need to put your efforts.
3. Causal Analysis
Causal analysis delves into the “cause and effect” relationship, aiming to understand why sales rise or fall. While time series forecasting focuses on past patterns, causal analysis explores the factors that trigger these changes.
How Causal Analysis Works: It examines both internal and external factors, such as economic conditions, competitor activity, or consumer preferences, and connects them to sales outcomes.
When to Use It: This model works best in environments where external factors significantly influence sales. Here’s an example to understand how to create a sales forecast using this model: a coffee chain noticing a dip in sales each January might find, through causal analysis, that colder weather and decreased consumer spending are contributing to the drop, prompting them to plan seasonal promotions or discounts.
4. Trend Analysis
Trend analysis zooms out to spot bigger shifts in sales over time, tracking steady rises or drops. Unlike time series, which focuses on short-term fluctuations, trend analysis captures the long game, revealing broader market movements.
How Trend Analysis Works: This model looks at data over extended periods, identifying gradual changes that may not be immediately obvious, such as a steady rise in product demand or the slow decline of a product category.
When to Use It: Trend analysis works best for businesses in stable industries where growth or decline is predictable. An example of a sales forecast using this model would be an eco-friendly brand using trend analysis to predict continued annual growth in demand for sustainable products. This enables them to plan for product expansion or increase manufacturing capacity to meet future demand.
5. Moving Average
The moving average model smooths out fluctuations in sales data to create a more stable forecast. It’s particularly useful when short-term changes or irregular events could distort predictions.
How Moving Average Works: The moving average calculates the average of a set number of data points over a specific period, such as average monthly sales over the past six months. This smooths out seasonal spikes or unexpected dips, providing a clearer view of the underlying trend.
When to Use It: Moving averages are ideal when sales data includes temporary fluctuations that do not reflect long-term demand, such as flash sales or holiday events. A clothing retailer, for example, might use this model to separate the effects of a seasonal discount from its regular sales patterns.
6. Econometric Modeling
Econometric modeling weaves together your sales data with larger economic trends—anything from inflation rates and interest rates to employment shifts. By doing so, it captures how big-picture economic shifts ripple down to impact sales performance on a granular level.
How Econometric Modeling Works: This model links your sales data to external economic indicators, translating changes in the economy to forecasted effects on your business. In practice, econometric modeling might reveal, for instance, that a downturn lowers consumer spending in key sectors, signaling to your team when sales may dip or surge.
When to Use It: Econometric modeling is key for sectors sensitive to economic changes—think luxury brands or automotive industries. A high-end car manufacturer, for example, could use it to understand how shifts in consumer confidence or interest rates will affect demand, helping them gauge when to ramp up or scale down production well in advance.
7. Machine Learning Models
Machine learning models go beyond traditional statistical methods by using algorithms to detect hidden patterns and refine predictions as they process more data. These models improve over time, making them highly adaptable.
How Machine Learning Works: These models sift through massive amounts of data, uncovering hidden connections, trends, or even things that don’t quite fit. The magic happens as they keep learning and adjusting, refining their predictions and offering a clearer picture of sales behavior as they go along.
When to Use It: These models are ideal for businesses in fast-paced, data-driven industries, like e-commerce, where large datasets and rapid changes in consumer behavior are common. For example, an online retailer might use machine learning to analyze purchase behavior and adjust pricing and inventory dynamically, factoring in everything from weather forecasts to browsing history.
8. Delphi Method
When there’s a lack of data or extreme market fluctuations, the Delphi method pulls in expert predictions to bridge the gaps. By tapping into the expertise of industry specialists, Delphi delivers more reliable sales forecasts based on first-hand insights.
How Delphi Works: This method uses a series of questionnaires sent to a group of experts, with responses reviewed and refined after each round. The experts revisit their predictions, adjusting based on the collective feedback until they arrive at a well-rounded forecast.
When to Use It: Delphi proves invaluable in markets with limited or rapidly changing data. For instance, a startup in the tech sector might lean on Delphi to gauge demand for an untested product, collecting valuable intel from industry veterans on trends, consumer interest, and potential adoption rates.
By embracing effective sales forecasting methods, from the analytical precision of time series models to the adaptive power of machine learning, businesses can predict trends, avoid pitfalls, and plan for success.
The best sales forecasting methods don’t just give you numbers—they give you insight, allowing you to steer your business with confidence through shifting markets.
Whether you’re optimizing inventory or planning marketing strategies, forecasting models for sales are your secret weapon, turning data into action and propelling you toward growth and sustainability.
An Improved Approach to Sales Forecasting
Sales forecasting is no longer reserved for the corporate giants. Small and mid-sized businesses can benefit from sales forecasting methods in sales management, streamlining decisions and enhancing growth.
Using effective techniques of sales forecasting gives you the foresight to keep your business on track. But how about dealing with all that data by hand? That can quickly feel like you’re drowning in spreadsheets.
That’s where Cash Flow Frog’s sales forecasting tool comes in. It simplifies the forecasting process by automating the data analysis, offering businesses an efficient and accurate way to predict sales without the complexity.
What are the methods of sales forecasting you're using to drive growth? We'd love to hear about your approach and how it's working for your business!
FAQ
Related posts:
You may be interested:
New:
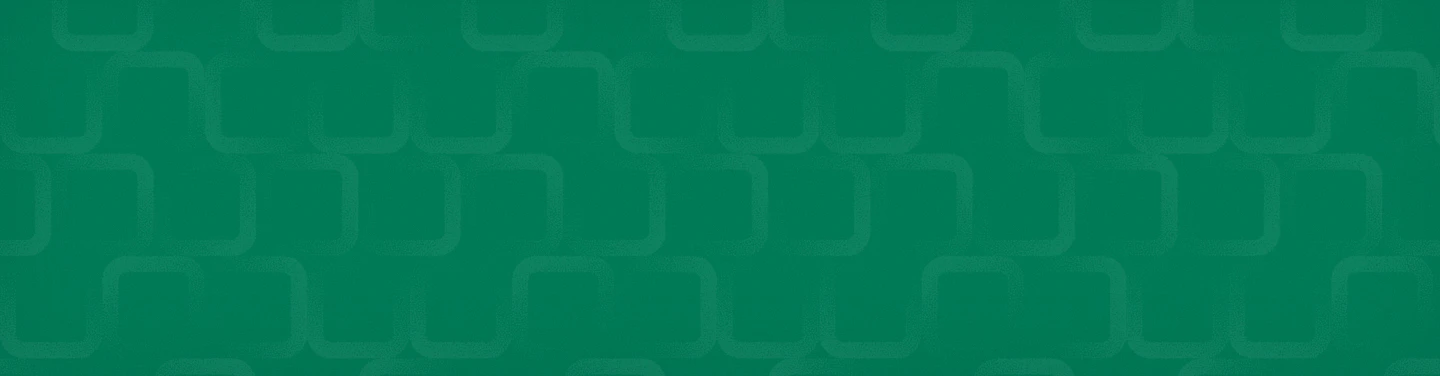